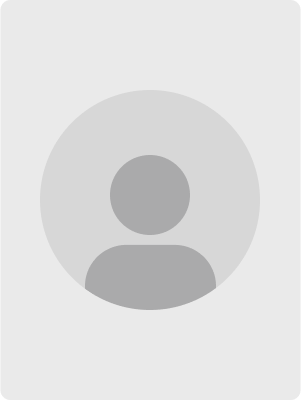
CAD system for automated colorectal polyp detection and characterisation (ColonCAD)
The ColonCAD project aims to develop an intelligent technological platform for the detection and classification of early signs of colon polyps, through the analysis and classification of images taken in real time during a colonoscopy. It is intended to design a CAD (Computer Aided Diagnosis) system based on different techniques of Artificial Intelligence and Images processing and analysis to classify the polyps into 5 classes: normal, hyperplasia, adenoma, superficial and infiltrative. The aim of this solution is to provide the doctor with a decision support solution, during the performance of a single colonoscopy exam, so that he can make the diagnosis in a more informed way and, if there are polyps that present characteristics that require its removal, that this operation take place during the same exam or that it is recommended that its removal be performed by surgery, when the nature of the polyp so indicates. This tool will avoid costs associated with polypectomies that are performed today and that, in view of their results, are considered as unnecessary. On the other hand, it will also be possible to minimize the number of tests to be carried out on the patient, avoiding subjecting him to the risk that these types of tests present for his health and future quality of life (for example, accidental colon perforation; endoscopically removing infiltrative lesions, which clinically should undergo surgery, among others).
Funding Agency
PORTUGAL 2020 – Sistema de Incentivos à Investigação e Desenvolvimento Tecnológico, para a execução do projeto I&DT Empresas em CoPromoção
Project Reference
POCI-01-0247-FEDER-047757
Project Members
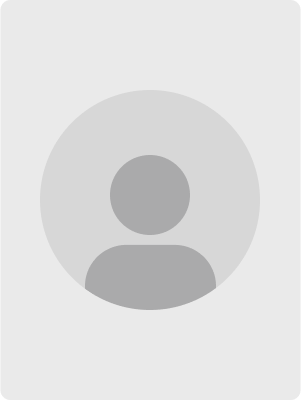
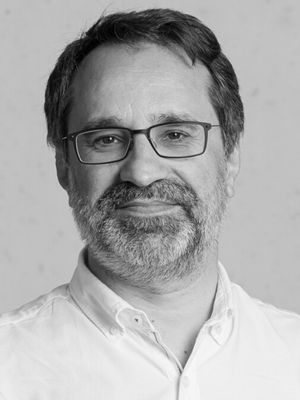
Patrício Costa
Main Project Outcomes
S. Queirós, “Right ventricular segmentation in multi-view cardiac MRI using a unified U-net model”, in E. Puyol Antón et al. (eds) Statistical Atlases and Computational Models of the Heart. Multi-Disease, Multi-View, and Multi-Center Right Ventricular Segmentation in Cardiac MRI Challenge. STACOM 2021. Lecture Notes in Computer Science, vol 13131, pp. 287-295, Springer, Cham, 2022.
“Best Paper Award in the M&Ms-2 Challenge”, by M&Ms2 Challenge organizers and the Medical Image Computing and Computer Assisted Intervention (MICCAI) Society.
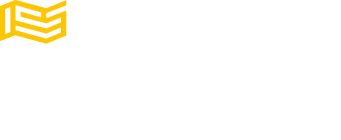
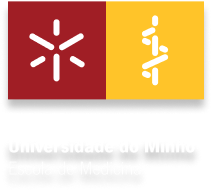

Contact us
Phone: +351 253 604 967
Fax: +351 253 604 809
Email: icvs.sec@med.uminho.pt
Address
Life and Health Sciences
Research Institute (ICVS)
School of Medicine,
University of Minho,
Campus de Gualtar
4710-057 Braga
Portugal

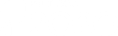
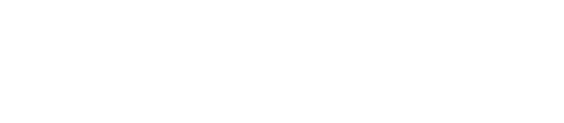
Copyright ©2022 ICVS. All Rights Reserved
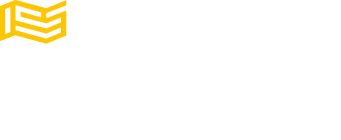
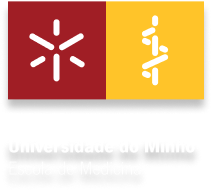

Copyright ©2022 ICVS. All Rights Reserved
Address
Life and Health Sciences
Research Institute (ICVS)
School of Medicine,
University of Minho,
Campus de Gualtar
4710-057 Braga
Portugal
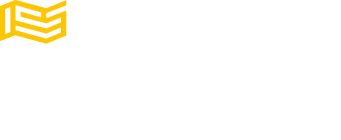

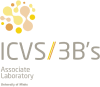
Copyright ©2022 ICVS. All Rights Reserved
Address
Life and Health Sciences
Research Institute (ICVS)
School of Medicine,
University of Minho,
Campus de Gualtar
4710-057 Braga
Portugal